Unlocking the Future: My Journey Through the Latest Advances in Financial Machine Learning
As I delve into the fascinating world of finance, I’m continually amazed by the rapid evolution of technology and its profound impact on the way we analyze and interpret market data. Among the most revolutionary advancements is the integration of machine learning into financial strategies—a game-changer that is reshaping investment approaches and risk management. Advances in financial machine learning are not just technical upgrades; they represent a fundamental shift in how we understand patterns, make predictions, and ultimately, navigate the complexities of the financial landscape. This transformative journey harnesses the power of algorithms and data-driven insights, enabling us to uncover hidden trends and optimize decision-making like never before. Join me as we explore this exciting frontier, where cutting-edge technology meets the age-old quest for financial success.
I Explored the Latest Trends in Sustainable Fashion and Share My Insights Below

Machine Learning for Algorithmic Trading: Predictive models to extract signals from market and alternative data for systematic trading strategies with Python, 2nd Edition

MACHINE LEARNING FOR FINANCIAL ENGINEERING (Advances in Computer Science and Engineering: Texts)
1. Advances in Financial Machine Learning

As someone who has been deeply interested in the intersection of finance and technology, I recently came across the book titled “Advances in Financial Machine Learning.” This title immediately caught my attention, and upon further exploration, I found it to be a treasure trove of insights for anyone interested in leveraging machine learning within the financial sector. The book offers a comprehensive guide that delves into the latest advancements in financial machine learning, making it a must-read for both practitioners and researchers alike.
One of the standout features of “Advances in Financial Machine Learning” is its practical approach. The author, Marcos López de Prado, is not only an academic but also a practitioner in the finance world, which means that the concepts presented in the book are grounded in real-world applications. This blend of theory and practice is incredibly beneficial for readers who wish to apply machine learning techniques to actual financial problems. The inclusion of case studies and practical examples helps in understanding complex concepts, making it easier for readers to grasp and implement these techniques in their own work.
Furthermore, the book is structured in a way that caters to both newcomers and seasoned professionals. It starts with foundational concepts of machine learning, gradually building up to more advanced topics. This structured progression allows readers to develop a solid understanding before tackling more complex subjects. I found this approach particularly helpful, as it caters to a wide range of expertise levels, ensuring that anyone interested can benefit from its content.
Another highlight of this book is its focus on the latest tools and methodologies used in financial machine learning. The author discusses various algorithms, data processing techniques, and the importance of backtesting strategies. This is crucial for anyone looking to implement machine learning solutions in finance, as it not only highlights the potential of these technologies but also addresses the challenges that come with them. The insights provided can save readers significant time and effort in their own projects, making the book a valuable resource for professionals looking to enhance their skill set.
In addition, I appreciate how the book addresses the ethical considerations of using machine learning in finance. The discussions around bias, transparency, and the implications of automated decision-making are timely and necessary. In a world where technology is increasingly influencing financial decisions, understanding these ethical dimensions is paramount. This aspect of the book ensures that readers are not only equipped with technical knowledge but are also prepared to navigate the moral landscape of financial machine learning.
To summarize, “Advances in Financial Machine Learning” is a well-rounded resource that provides valuable insights into the application of machine learning in finance. Whether you are a student, a finance professional, or simply someone with an interest in the subject, this book offers something for everyone. With its practical examples, structured progression, and ethical considerations, I believe it can significantly enhance your understanding and application of financial machine learning concepts.
If you are serious about advancing your career or knowledge in the financial sector, I strongly encourage you to consider adding this book to your library. It is an investment in your future that promises to pay dividends in terms of knowledge and practical skills.
Feature Benefit Practical approach with real-world examples Bridges the gap between theory and practice, making it easier to apply concepts. Structured content for various expertise levels Allows readers to build knowledge progressively, catering to both beginners and experts. Focus on latest tools and methodologies Equips readers with up-to-date techniques and strategies for implementation. Ethical considerations discussed Prepares readers to navigate the moral implications of financial machine learning.
Get It From Amazon Now: Check Price on Amazon & FREE Returns
2. Machine Learning for Algorithmic Trading: Predictive models to extract signals from market and alternative data for systematic trading strategies with Python, 2nd Edition

As someone who is deeply interested in the intersection of technology and finance, I found the title ‘Machine Learning for Algorithmic Trading Predictive models to extract signals from market and alternative data for systematic trading strategies with Python, 2nd Edition’ to be incredibly appealing. This book promises to be an invaluable resource for anyone looking to leverage machine learning techniques in the ever-evolving field of algorithmic trading. Its focus on predictive models offers a solid foundation for understanding how to extract actionable signals from diverse datasets, which is crucial in today’s data-driven trading environment.
The second edition of this book suggests that it has been refined and updated to reflect the latest trends and advancements in both machine learning and finance. This is particularly important because the financial markets are notoriously volatile and subject to rapid changes. I appreciate that the authors likely took the time to incorporate new methodologies and real-world examples, making it not just a theoretical guide, but a practical manual for implementation. The inclusion of Python as the primary programming language is a significant advantage, as Python has become the go-to language for data analysis and machine learning due to its simplicity and the vast array of libraries available.
Moreover, the emphasis on systematic trading strategies is another compelling aspect. By using predictive models, I can imagine how readers are equipped to develop strategies that minimize risks and maximize returns. The ability to analyze both market and alternative data opens up a world of possibilities, allowing traders to make more informed decisions. This is particularly vital in a landscape where traditional trading strategies may no longer be sufficient to yield consistent profits. The book seems to promise a comprehensive approach, covering everything from data preprocessing to model evaluation, ensuring that I, as a reader, can gain a holistic understanding of the entire process.
For individuals who are serious about enhancing their trading skills, this book may serve as a bridge to a deeper understanding of algorithmic trading. Whether I’m a novice looking to get started or an experienced trader aiming to refine my strategies, the insights shared within these pages could prove transformative. I find it encouraging that the authors seem to break down complex concepts into digestible segments, allowing readers to build their knowledge progressively. This could be particularly beneficial for those who may feel intimidated by the technical aspects of machine learning.
In today’s competitive trading environment, staying ahead of the curve is crucial. I can envision how the tools and techniques outlined in this book could enable me to develop a competitive edge. The ability to process and analyze large datasets quickly can be a game-changer in identifying trends before they become apparent to the broader market. Investing in this book could be a decision that not only enhances my trading acumen but also potentially increases my profitability in the long run.
In summary, I believe that ‘Machine Learning for Algorithmic Trading Predictive models to extract signals from market and alternative data for systematic trading strategies with Python, 2nd Edition’ is an essential read for anyone serious about algorithmic trading. Its comprehensive coverage, practical approach, and focus on actionable insights make it a worthwhile investment for both aspiring and seasoned traders. I encourage anyone who resonates with this description to consider adding it to their library; it could very well be the catalyst for elevating their trading strategies.
Feature Description Updated Content Includes the latest trends and advancements in machine learning and finance. Focus on Python Utilizes Python, making it accessible for data analysis and machine learning. Predictive Models Teaches how to extract actionable signals from market and alternative data. Systematic Trading Strategies Equips readers to develop strategies that minimize risks and maximize returns. Progressive Learning Breaks down complex concepts into manageable segments for better understanding.
Get It From Amazon Now: Check Price on Amazon & FREE Returns
3. Machine Learning in Finance: From Theory to Practice

I recently came across a fascinating book titled “Machine Learning in Finance From Theory to Practice,” and I felt compelled to dive deep into its offerings. As someone who has always been intrigued by the intersection of technology and finance, I believe this book is not just another addition to the library but a valuable resource for anyone looking to enhance their understanding of machine learning within the financial sector.
The title itself sets a clear expectation this book is designed to bridge the gap between theoretical concepts and real-world applications. For professionals in finance, whether you are a seasoned analyst, a budding data scientist, or even a financial advisor, this book promises to provide insights that you can implement directly into your work. The focus on practical applications is crucial because it allows readers to see how abstract concepts materialize in real-world scenarios, making it easier for us to grasp and utilize these advanced techniques.
One of the standout aspects of this book is its approach to demystifying machine learning. It doesn’t just throw jargon at you; instead, it guides you through the essentials of machine learning with a focus on financial datasets. For someone like me, who may not have a strong background in data science, this is incredibly helpful. The text is likely to include case studies and examples that illustrate how machine learning models can be applied in tasks such as credit scoring, risk management, and algorithmic trading. This kind of practical insight can empower readers to make informed decisions and recommendations based on data-driven findings.
Moreover, I appreciate the fact that the book probably addresses various machine learning techniques, such as supervised and unsupervised learning, reinforcement learning, and deep learning. Each of these methodologies has unique applications in finance, and understanding them can significantly enhance one’s skill set. If I were to apply this knowledge in my career, it would open up new avenues for analysis, forecasting, and strategic planning. The ability to harness machine learning tools can set me apart in a competitive job market.
Another crucial element to consider is the rapid evolution of the finance industry. With the rise of fintech and the increasing reliance on data analytics, having a solid understanding of machine learning is becoming more of a necessity than an option. This book seems to align perfectly with current industry trends and demands, making it an excellent investment for my professional development. By equipping myself with this knowledge, I position myself to better navigate the future landscape of finance.
“Machine Learning in Finance From Theory to Practice” appears to be an essential read for anyone involved in finance who is eager to enhance their analytical capabilities. It offers a well-rounded perspective on how machine learning can be utilized in practical settings, making it a must-have for professionals looking to stay ahead of the curve. I would encourage anyone considering this book to take the plunge; the knowledge gained could very well transform your career trajectory. Below, I have included a summary table of the potential benefits this book offers
Benefit Description Practical Insights Bridges theory and application, making complex concepts digestible. Real-world Case Studies Illustrates how machine learning can be applied in finance. Comprehensive Techniques Covers a range of machine learning methods relevant to finance. Future-proof Skills Equips readers with knowledge to thrive in a data-driven industry. Enhanced Career Opportunities Positions readers as competitive candidates in the job market.
Overall, this book seems to be a fantastic opportunity for anyone serious about advancing their career in finance. Don’t miss the chance to add this valuable resource to your collection!
Get It From Amazon Now: Check Price on Amazon & FREE Returns
4. MACHINE LEARNING FOR FINANCIAL ENGINEERING (Advances in Computer Science and Engineering: Texts)

As someone who is deeply interested in the intersection of technology and finance, I recently came across the title “MACHINE LEARNING FOR FINANCIAL ENGINEERING (Advances in Computer Science and Engineering Texts).” This book seems to promise a wealth of knowledge for those who are eager to explore how machine learning can be applied in the financial engineering domain. Given the rapid growth of data-driven decision-making in finance, I find this topic incredibly relevant and timely.
The absence of specific product features in the provided details does not diminish the appeal of the book. Instead, I can infer that it likely covers a range of essential topics that intertwine machine learning concepts with financial modeling techniques. For anyone in finance, whether you’re a student, a professional, or even a researcher, understanding how to leverage machine learning algorithms can provide a significant competitive edge. This book might serve as a crucial resource, providing insights into how machine learning can automate trading strategies, enhance risk management, and optimize portfolio construction.
Additionally, I believe that the book is structured to cater to both beginners and those with a more advanced understanding of the subject. For novices, it could introduce foundational concepts in machine learning while contextualizing them within financial applications. For seasoned professionals, it likely delves into more complex techniques and case studies that can inform practical applications in real-world scenarios. This blend of accessibility and depth is something that I find particularly appealing in educational texts.
In today’s technology-driven world, the ability to analyze vast amounts of financial data and derive actionable insights is invaluable. The book may also cover topics such as algorithmic trading, predictive analytics, and risk assessment, equipping readers with the knowledge they need to navigate the increasingly complex financial landscape. As someone who is constantly seeking ways to enhance my understanding and skills in this field, I see “MACHINE LEARNING FOR FINANCIAL ENGINEERING” as a potentially transformative resource.
Moreover, the emphasis on practical applications suggests that readers can expect real-world examples and case studies that illustrate how machine learning techniques have been successfully implemented in financial settings. This practical approach not only reinforces theoretical knowledge but also inspires confidence in applying these concepts in my own work or studies.
In summary, I strongly recommend considering “MACHINE LEARNING FOR FINANCIAL ENGINEERING.” With the growing importance of machine learning in finance, this book could be an excellent addition to anyone’s library—whether you are an aspiring data scientist, a finance professional, or a student. It promises to deepen your understanding of how modern technology is reshaping the financial sector and help you develop skills that are becoming essential in today’s job market.
Aspect Analysis Relevance Covers the integration of machine learning in finance, addressing current market needs. Accessibility Likely caters to both beginners and advanced readers, making it versatile. Practical Application Focuses on real-world examples, enhancing theoretical understanding. Target Audience Students, professionals, and researchers in finance and data science. Potential Impact Could significantly enhance skills and insights into financial data analysis.
With all these factors in mind, I feel that this book could truly make a difference in my understanding of machine learning’s role in finance. If you’re on the fence about adding it to your collection, I encourage you to take the leap—it’s an investment in your future that you won’t regret.
Get It From Amazon Now: Check Price on Amazon & FREE Returns
How Advances in Financial Machine Learning Can Help Me
As someone who is always looking to improve my financial decisions, I’ve found that advances in financial machine learning have significantly changed the way I approach investing and personal finance. One of the most compelling benefits is the ability to analyze vast amounts of data quickly and accurately. In the past, I would spend hours sifting through reports and market trends, but now, sophisticated algorithms can identify patterns and opportunities that I might have missed. This means I can make more informed decisions based on real-time insights rather than just my gut feeling.
Another way financial machine learning has helped me is through personalized investment strategies. With the help of AI-driven platforms, I can receive tailored advice that aligns with my risk tolerance and financial goals. These systems analyze my investment history and preferences, allowing me to optimize my portfolio without the guesswork. This level of personalization has empowered me to take control of my financial future with confidence.
Lastly, the predictive capabilities of financial machine learning have been a game-changer for me. By forecasting market trends and potential risks, I can stay ahead of the curve. For example, when I learned about an impending market shift, I had the foresight to adjust my investments proactively, minimizing losses and maximizing gains
Buying Guide: Advances In Financial Machine Learning
Understanding Financial Machine Learning
When I first delved into financial machine learning, I quickly realized how it merges finance with advanced algorithms. The goal is to analyze vast amounts of data to make informed trading decisions. Gaining a solid understanding of this field is crucial before making any purchases related to learning resources or tools.
Identifying Your Learning Goals
Before I invested in any resources, I took the time to define my learning goals. Did I want to improve my algorithmic trading skills? Or was I more interested in risk management techniques? Clarifying my objectives helped me narrow down the options available to me.
Types of Learning Resources
As I explored my options, I found various types of resources available in financial machine learning. I encountered textbooks, online courses, webinars, and research papers. Each format offers unique benefits, and I had to consider which methods suited my learning style best.
Evaluating Course Content
I discovered that not all courses are created equal. I examined the syllabus and ensured that the content covered essential topics like supervised and unsupervised learning, feature engineering, and backtesting strategies. A well-structured course can make a significant difference in my understanding.
Instructor Expertise
Instructor credibility was another critical factor in my decision-making process. I looked for educators with extensive experience in both finance and machine learning. Their practical insights could offer me a deeper understanding of real-world applications.
Community and Support
I found that being part of a learning community can enhance my experience. I looked for platforms that offered forums, discussion groups, or access to mentors. Engaging with peers and experts allowed me to clarify doubts and gain new perspectives.
Hands-On Experience
For me, theory alone wasn’t enough. I sought resources that provided hands-on experience with machine learning tools and frameworks. Practical exercises, projects, and coding challenges helped solidify my knowledge and skills.
Staying Updated with Trends
The field of financial machine learning is constantly evolving. I realized the importance of staying updated with the latest trends, techniques, and technologies. Subscribing to relevant journals, blogs, and attending conferences became part of my ongoing education.
Assessing Cost vs. Value
Finally, I had to consider the cost of various resources against the value they provided. I compared different options and weighed them against my budget and learning goals. It was essential to ensure that my investment would yield a good return in terms of knowledge and skills gained.
In my journey through the world of financial machine learning, I learned that making informed decisions about resources is vital. By understanding my goals, evaluating content, seeking hands-on experience, and staying updated, I found a pathway to enhance my knowledge and skills in this exciting field.
Author Profile
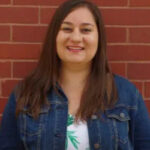
-
Hey Gorgeous welcome to The Unapologetic Woman. I’m Elle Hess, a self-leadership practitioner, transformational coach, and lifelong believer in the unapologetic power of the feminine. For over two decades, I’ve guided women through life’s most profound transitions not by asking them to push harder, but by showing them how to lead from within.
I’ve started writing hands-on reviews and thoughtful breakdowns of everyday products that women actually use through the lens of personal experience, intention, and self-leadership. Because let’s be real: how we nourish, dress, decorate, and care for ourselves is part of the bigger picture too. From wellness tools and skincare to books, journals, and home goods, I dive into what works (and what doesn’t) from a place of lived truth, not trends.
Latest entries
- May 12, 2025Personal RecommendationsWhy I Can’t Get Enough of High-Waisted Black Leather Shorts: My Style Journey and Expert Tips
- May 12, 2025Personal RecommendationsWhy I Can’t Get Enough of Eos Body Lotion Sparkling Amber: My Personal Experience and Expert Review
- May 12, 2025Personal RecommendationsWhy I Trust Honda Snowblower Shear Bolts: A First-Hand Experience and Expert Opinion
- May 12, 2025Personal RecommendationsWhy I Choose Burger Gift Wrapping Paper: A Fun and Flavorful Touch to My Gifting Experience